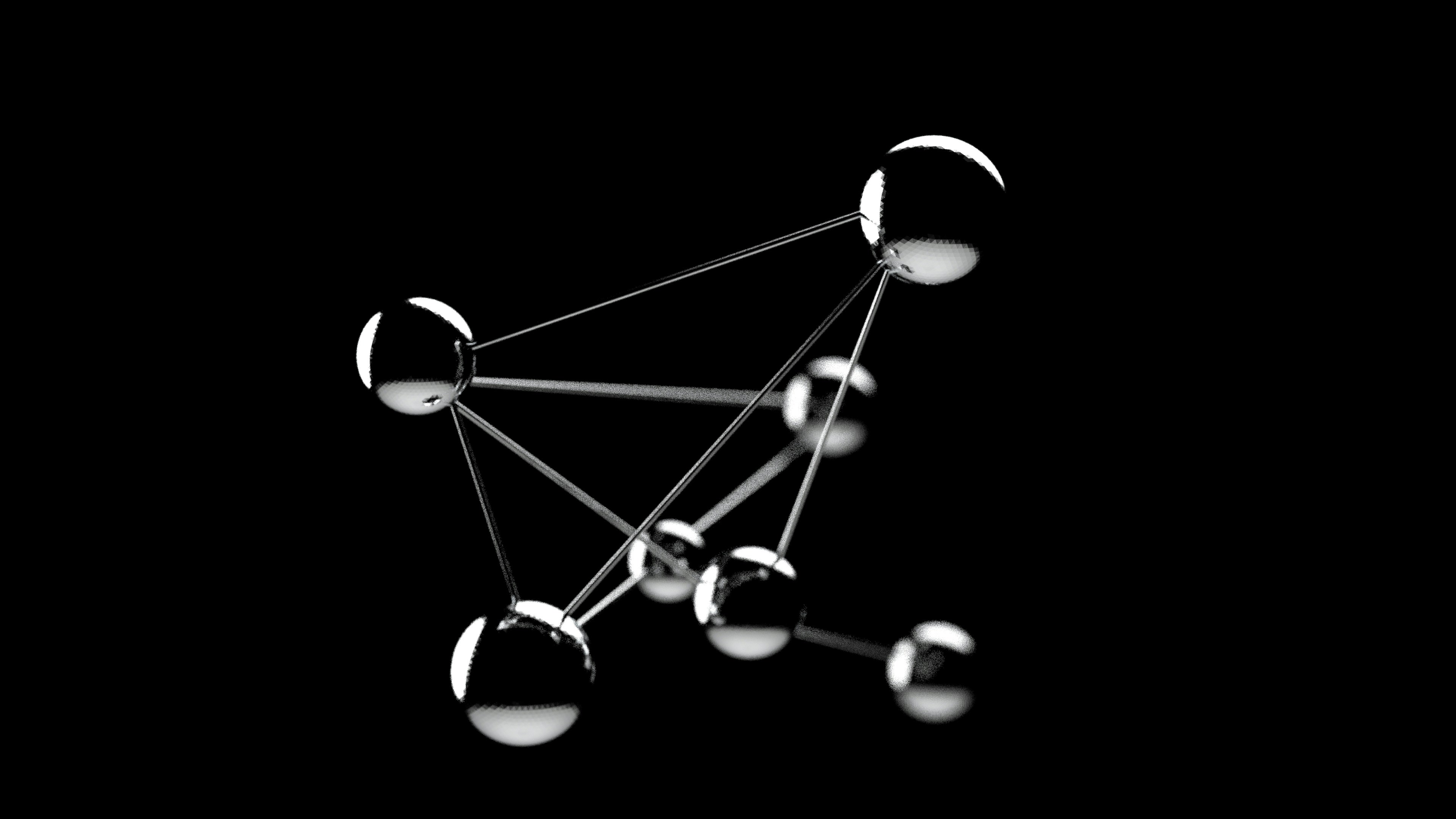
Quantum-Enhanced AI in Space—Propelling the Next Frontier of Exploration
Space exploration has long been a showcase of human ingenuity—launching satellites that enable global connectivity, sending probes to other planets, and even eyeing the possibility of interplanetary travel. Yet, as missions become more complex—think long-duration travel, real-time data analysis from distant probes, and advanced Earth observation—traditional computing methods can struggle to handle the colossal volumes of data and the intricate physics involved. Artificial Intelligence (AI) has helped automate some decision-making (like autonomous rovers on Mars), but the tasks ahead—such as on-orbit servicing, space debris management, or deep-space exploration—demand a new tier of computational capability.
Enter quantum computing, a revolutionary technology that harnesses phenomena like superposition and entanglement to perform certain computations at speeds unimaginable on classical hardware. By merging quantum hardware with AI—often termed quantum-enhanced AI—the space sector stands to overcome limitations in orbital mechanics, big-data analytics, and complex resource optimisation. From more efficient satellite constellations to cutting-edge astrophysical research, this synergy could herald a new era of space exploration and commercial ventures.
In this article, we will:
Survey the current challenges in space-based missions, from planning and operations to data processing.
Demystify quantum computing—how qubits differ from bits and why quantum algorithms can outperform classical ones in specific contexts.
Examine how quantum-enhanced AI can transform various space applications, such as satellite fleets, deep-space comms, Earth observation, and more.
Identify the main roadblocks (hardware maturity, data transfer) and how the industry might address them.
Highlight new career pathways, skills, and roles emerging at the crossroads of space technology, AI, and quantum computing—particularly in the UK.
Whether you’re a satellite engineer, astrophysicist, data scientist, or a space enthusiast curious about where technology is heading, read on. Quantum-enhanced AI may become the linchpin for the next wave of advances in space exploration, driving more efficient missions, groundbreaking research, and a thriving job market.
1. The Space Sector’s Growing Demands
1.1 Major Achievements and Ongoing Expansion
Satellites power our communications, navigation (GPS/Galileo), and Earth observation (weather, climate tracking). Space agencies have landed rovers on Mars, studied near-Earth asteroids, and even tested the feasibility of commercial spaceflight. Meanwhile:
Mega-Constellations: Thousands of small satellites in low Earth orbit (LEO) deliver global broadband (e.g., Starlink, OneWeb).
Commercial Launch Providers: Private companies now vie with government agencies, lowering launch costs and increasing flight frequency.
Deep-Space Exploration: Missions like ESA’s JUICE (Jupiter Icy Moons Explorer) or NASA’s Artemis highlight renewed interest in outer solar system travel and human return to the Moon.
Yet complexity is skyrocketing—constellations produce vast telemetry data, deep-space probes must navigate harsh cosmic conditions, and emerging “NewSpace” markets push for more agile mission design.
1.2 Challenges with Classical Computing
Mission Planning & Optimisation: Scheduling satellite contacts, planning flight trajectories, or coordinating multi-spacecraft missions is often a combinatorial puzzle that overwhelms classical methods.
On-Board Processing: Spacecraft generally have power and cooling constraints, limiting the sophistication of AI hardware in orbit.
High-Dimensional Data: Earth observation satellites capture streams of high-resolution, multi-spectral imagery, which can swamp classical analytics if near-real-time insights are required.
Deep-Space Delays: Long communication latencies (minutes to hours) complicate live analytics or reactivity, emphasising the need for powerful autonomous decision-making.
Quantum-enhanced AI offers potential accelerations for path planning, large-scale data analytics, and in-flight autonomy—if hardware and integration hurdles can be surmounted.
2. Quantum Computing Essentials
2.1 Qubits vs. Bits
Classical machines use bits (0 or 1). Quantum computers use qubits:
Superposition: A qubit can occupy a combination of 0 and 1 simultaneously, enabling parallel computations over many states.
Entanglement: Qubits can be intertwined so that measuring one affects the other, providing computational correlations unattainable with classical bits.
2.2 The NISQ Era
Currently, we’re in the NISQ (Noisy Intermediate-Scale Quantum) era, where quantum devices:
Have Limited Qubits: Tens or low hundreds, prone to noise.
Short Coherence Times: Qubit states degrade quickly, restricting the complexity and duration of computations.
Are Mostly Cloud-Based: IBM, Google, Microsoft, Amazon, and start-ups offer remote quantum hardware access, but dedicated on-site quantum machines remain rare.
Despite these limitations, quantum computers already show promise in solving or speeding up certain classes of problems relevant to space missions.
3. Quantum-Enhanced AI: Why It Matters in Space
3.1 The Fusion of Two Cutting-Edge Technologies
Quantum-enhanced AI merges quantum algorithms with machine learning frameworks to potentially deliver:
Faster Training & Inference: Offloading resource-intensive computations (like large-scale optimisation or certain matrix operations) to quantum hardware.
New ML Architectures: Quantum Neural Networks (QNNs) might detect complex relationships in high-dimensional cosmic or Earth-observation data.
Improved Optimisation & Sampling: Large-scale route planning, sensor scheduling, or constellation design could benefit from quantum’s parallel searching capabilities.
3.2 Hybrid Workflows
Because quantum hardware is limited, real-world solutions adopt hybrid approaches:
Classical HPC or On-Board Compute: Manages data ingestion and standard ML tasks.
Quantum Subroutines: Specific tasks (like advanced optimisation or sampling) offloaded to quantum systems.
Integration: Outputs feed into the overall mission control, ground station analytics, or on-board autonomy loop.
This mirrors how GPUs accelerate deep learning on Earth—only here, the co-processor is a quantum device, typically accessed via secure network links.
3.3 Transforming Space Missions
Whether boosting on-board autonomy for interplanetary probes, orchestrating thousands of satellites, or analysing giant streams of remote sensing data, quantum-enhanced AI could redefine efficiency and capability, propelling the industry past existing computational walls.
4. Use Cases: Quantum-Enhanced AI in Space
4.1 Satellite Constellation Management
Mega-constellations can involve thousands of satellites:
Orbital Slot Optimisation: Minimising collision risks, orbital congestion, and service overlap is a combinatorial challenge. Quantum algorithms (QAOA) might quickly converge on near-optimal configurations.
Spectrum Allocation & Inter-Satellite Comms: Assigning frequencies or time slots to reduce interference benefits from advanced scheduling.
Reconfiguration & Collision Avoidance: If satellites fail or debris threatens an orbit, quantum-based planning could recalculate new orbits fast.
4.2 Interplanetary Trajectory Design
Deep-space missions require intricate gravitational assists and fuel constraints:
Gravity-Assisted Path Planning: Evaluate thousands of potential flyby sequences (like multiple planet slingshots) faster, saving mission planners weeks of HPC.
Landing on Small Bodies: Quantum-based trajectory analysis may refine approach vectors under complex gravity fields.
Multi-Objective Optimisation: Balancing flight time, fuel consumption, and scientific return can be tackled by quantum subroutines for advanced mission design.
4.3 On-Board Data Processing and Autonomy
Satellites, rovers, and probes gather enormous volumes of scientific data:
Quantum-Assisted Image Analysis: Quickly classify or compress imagery (e.g., from rovers or telescopes) before transmitting to Earth, saving bandwidth.
Sensor Fusion for Landers/Orbiters: Real-time integration of radar, lidar, and visual data for hazard detection or sample targeting. Quantum ML might handle high-dimensional streams more efficiently.
Autonomous Navigation: Deep-space probes or planetary rovers with quantum-based reinforcement learning adapt to unknown terrain or dynamic events (dust storms, meteorite hits) in near real-time.
4.4 Earth Observation and Climate Monitoring
Satellites monitoring Earth produce multi-spectral, high-resolution data:
Quantum-Accelerated Image Classification: Identify deforestation, crop health, or pollution hotspots across petabytes of data.
Extreme Weather Prediction: Combining cosmic ray data, atmospheric variables, and machine learning can yield advanced forecasting. Quantum sampling helps handle chaotic, high-dimensional models.
Rapid Disaster Response: AI-powered analytics for floods, hurricanes, or fires is sped up with quantum subroutines, guiding relief efforts in real-time.
4.5 Space Debris Tracking and Removal
Low Earth orbit is crowded with junk. Collision risk is high:
Quantum-Assisted Debris Mapping: Master huge ephemeris data to predict close passes or collisions.
Optimised Debris Removal Missions: Scheduling removal sequences for multiple debris objects in minimal time. Quantum search can handle multi-target route complexities.
5. Obstacles & Challenges
5.1 Hardware Maturity
Quantum computing is still NISQ:
High Noise & Short Coherence: Restricts the complexity of feasible algorithms.
Limited On-Orbit Quantum Solutions: Running quantum computations in space is far off, so tasks typically rely on Earth-based quantum servers.
Latency for Real-Time Missions: Long-distance comms with Earth-based quantum hardware might be impractical for certain time-sensitive tasks (especially deep-space missions).
5.2 Data Transfer and Encoding
Space missions can involve large data sets:
Bandwidth Constraints: Transmission from deep space orbits is slow, so sending entire data sets to quantum cloud might be infeasible.
Encoding Overhead: Encoding high-dimensional telemetry or sensor data into qubit states can overshadow potential gains if not carefully optimised.
5.3 Reliability and Safety
Space missions often have zero margin for error:
Mission-Critical Validation: Any quantum-based system or subroutine must undergo rigorous testing, meeting safety and redundancy standards.
Regulatory Oversight: ESA, NASA, and other agencies require proven reliability. Early quantum solutions might face skepticism until thoroughly validated.
5.4 Skills & Collaboration
Cross-Disciplinary Expertise: Bridging quantum computing, AI, and space engineering is extremely niche.
Shared Intellectual Property: Collaborations between space agencies, quantum start-ups, and AI firms demand careful IP agreements and data handling protocols.
6. Building Quantum-Enhanced Space Pipelines
6.1 A Typical Hybrid Workflow
Mission Data Capture: Satellite or rover collects raw data (images, sensor logs, orbital parameters).
Preprocessing / Edge Analysis: On-board or near real-time ground HPC filters and summarises data.
Quantum Task Offload: For tasks like advanced trajectory re-optimisation, large-scale image classification, or multi-satellite scheduling, data is securely transmitted to quantum cloud services.
Integration: Quantum outputs feed back into mission control or on-board flight software, guiding next steps or generating new insights.
Iterative Refinement: As conditions change (orbital dynamics, environmental factors), the loop repeats.
6.2 Tools and Platforms
Quantum SDKs: Qiskit (IBM), Cirq (Google), PennyLane (Xanadu) integrate with classical ML for building quantum circuits and models.
Space Agency Suites: NASA, ESA, UKSA might incorporate quantum modules into mission planning software or HPC clusters.
ML Frameworks: TensorFlow, PyTorch with quantum extensions (TensorFlow Quantum, PennyLane).
Cloud Infrastructures: AWS, Azure, Google Cloud offer HPC + quantum combos, potentially linking to existing aerospace data pipelines.
6.3 Best Practices
Identify Bottlenecks: Target quantum subroutines at the biggest HPC pain points—like route planning or big-data classification.
Prototype on Simulators: Test quantum approaches thoroughly via simulators before incurring cost/noise constraints of real hardware.
Iterate and Validate: Evaluate whether quantum subroutines deliver tangible speed-ups or accuracy benefits over classical HPC. Publish or share findings for community feedback.
7. Emerging Careers at the Quantum-Space Intersection
7.1 Quantum Space Mission Engineer
Algorithm Development: Adapting quantum computing solutions for mission-critical tasks (trajectory design, scheduling).
Software Integration: Embedding quantum calls into standard ground control or on-board flight software.
Research & Prototyping: Testing feasibility with mission simulators, HPC labs, or real flight experiments.
7.2 AI Developer (Quantum Focus) in Aerospace
ML Workflow Construction: Building hybrid models for satellite imagery analysis, route planning, or rover autonomy.
Cloud & DevOps: Managing quantum/hybrid pipelines, automating deployment, and verifying performance.
Benchmarking: Continuously comparing quantum vs. classical HPC results on real mission data sets.
7.3 Quantum Materials Scientist (Space Tech)
Advanced Materials Simulation: Using quantum chemistry for next-gen space materials (radiation shielding, lightweight composites).
Thermal & Structural Analysis: Incorporating quantum insights into spacecraft design at the molecular level.
Cross-Sector Collaboration: Working with aerospace component manufacturers to refine processes or discover new alloys.
7.4 Quantum Cryptography Specialist for Space
Satellite Link Security: Developing post-quantum cryptographic methods to secure satellite uplinks, TT&C (telemetry, tracking & command).
Quantum Key Distribution (QKD): Possibly implementing space-based QKD satellites, ensuring quantum-safe global communications.
Compliance & Standards: Guiding governments and agencies on quantum-secure protocols across international networks.
8. Ethical & Practical Considerations
8.1 Resource Allocation
Space missions are budget-sensitive. Quantum computing services remain costly:
ROI & Justification: Agencies and companies must see clear mission improvements before committing.
Sustainability: High-energy data centres for quantum might offset the green benefits of certain Earth observation or climate missions.
8.2 Data Handling & Sovereignty
Space data often has strategic or commercial value:
Encryption & Access Control: Must be robust, especially if using public cloud quantum services.
International Collaboration: Missions can be multinational, complicating data sharing and IP constraints.
8.3 Technological Dependence
Relying heavily on quantum cloud could introduce single points of failure or control. Redundancy or fallback classical approaches remain essential if quantum resources become unavailable.
8.4 Bias & Representation in AI Models
AI-driven decisions about climate interventions, resource allocation (like orbital slot assignment), or Earth observation tasks must remain equitable and transparent, not biased towards wealthier nations or specific commercial interests.
9. Vision: 1, 5, and 10 Years Ahead
9.1 Near-Term (1–2 Years)
Pilot Projects: R&D labs (public and private) run small-scale quantum trials, e.g., scheduling satellite passes or re-optimising flight trajectories.
Refined SDKs: Tools from IBM, Google, Microsoft, etc., expand quantum libraries tailored for aerospace and Earth observation.
Skill-Building & Events: Hackathons, workshops, and academic courses bridging quantum computing, AI, and space engineering.
9.2 Mid-Term (3–5 Years)
Partial Operational Use: Space agencies or large constellation operators adopt quantum subroutines for niche but critical tasks—like multi-satellite collision avoidance or advanced Earth imagery analysis.
Improved Quantum Hardware: Systems with hundreds or thousands of qubits, partial error correction, enabling moderate-scale space-related computations.
Standards & Frameworks: Agencies (ESA, NASA) introduce guidelines for quantum-based mission planning or cryptography, easing adoption.
9.3 Long-Term (5–10+ Years)
Mainstream Quantum-Enabled Missions: Large chunks of mission design, scheduling, and onboard analytics incorporate quantum-accelerated AI.
Advanced Autonomy in Deep Space: Rovers, orbiters, or crewed spacecraft rely heavily on quantum-driven decisions to handle unknown hazards or adapt long flight routes.
Revolutionary Discoveries & Data Insights: Quantum-level simulation fosters breakthroughs in astrophysics, planetary science, and near-real-time climate interventions.
10. Conclusion
Space exploration and satellite services have charted remarkable progress, but the future beckons with even more ambitious missions—sending robotic explorers to distant worlds, deploying mega-constellations to blanket Earth in connectivity, and safeguarding our orbital environment. Quantum computing, though still in its early stages, stands as a potential game-changer in tandem with AI. This quantum-enhanced synergy can tackle massive scheduling, route optimisation, or big-data analytics tasks that choke classical HPC solutions, enabling next-level autonomy and scientific insight.
Yet realising this vision will require interdisciplinary collaboration, from quantum algorithm experts to aerospace engineers and AI developers. As hardware matures and pilot projects validate quantum advantages, we can expect far-reaching impacts—faster mission planning, safer orbital operations, deeper planetary insights, and even new material discoveries for spacecraft design.
For professionals or aspiring talent seeking to shape the future of space technology—be it in satellite operations, mission control, or R&D labs—these developments open unique pathways. If you’re eager to explore exciting roles or stay ahead in the UK’s burgeoning space sector, visit www.ukspacejobs.co.uk. There, you’ll find opportunities at the cutting edge of aerospace innovation, including positions blending quantum computing, AI, and space engineering. Join the quest to expand our cosmic horizons—one quantum-enhanced step at a time.